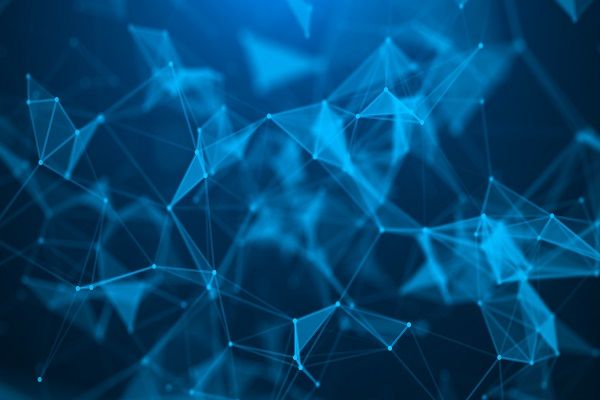
L’importanza dei dati nell’era 4.0. Imparare a riconoscere i Dark data: la bibliografia
Pubblichiamo di seguito la bibliografia completa dell’articolo L’importanza dei dati nell’era 4.0. Imparare a riconoscere i Dark data scritto da Vito Del Vecchio, Manuela Marra, Anna Maria Crespino e Mariangela Lazoi sul numero di febbraio 2020 di Sistemi&Impresa.
Banafa A. (2015), Understanding Dark data, https://www.bbvaopenmind.com/en/technology/digital-world/understanding-dark-data.
Cui Y., Sami K., Ka C. C., (2019), Manufacturing Big data ecosystem: a systematic literature review, Robotics and computer integrated manufacturing, 62, 101861.
Dean A. (2014), Applied predictive analytics. Principles and techniques for the professional data analyst, Wiley.
DELL Technologies Corporation, Digital universe invaded by sensors, 7th annual study reveals that digital universe to grow 10x by 2020, Data generated by sensor-enabled “things” to represent 10%, https://corporate.delltechnologies.com/en-us/newsroom/announcements/2014/04/20140409-01.htm, 2014.
Gandomi A., Haider M. (2015), Beyond the hype: Big data concepts, methods, and analytics, International journal of information management, 137-144.
Gartner (2019), Information Technology Gartner glossary, https://www.gartner.com/en/information-technology/glossary, 2019.
IBM, IBM Connects (2015), Internet of Things to the Enterprise, https://www-03.ibm.com/press/us/en/pressrelease/46453.wss.
Intel (2018), Datumize and Intel Transform Dark data into operational insight for manufacturing and logistics, https://software.intel.com/en-us/articles/datumize-and-intel-transform-dark-data-into-operational-insight.
Kusiak A.(2017), Smart manufacturing must embrace Big data, Nature – international weekly journal of science, 23-25.
McAfee A., Brynjolfsson E. (2012), Big data: the management revolution, https://hbr.org/2012/10/big-data-the-management-revolution.
McKinsey&Company (2016), The age of Analytics: competing in a Data-driven world, McKinsey Global Institute, https://it.scribd.com/document/362692385/MGI-the-Age-of-Analytics-Executive-Summary, 2016.
Md Ajis A.F., Baharin S. Hajar (2019), Dark data management as frontier of information governance, 9th IEEE symposium on computer applications and industrial electronics, 34-37. DOI 10.1109/ISCAIE.2019.8743915.
Miller G.J. (2019), A conceptual framework for interdisciplinary decision support project success, IEEE Technology & Engineering management conference (TEMSCON), Heidelberg, Germany.
Nedelcu B.(2013), About Big data and its challenges and benefits in Manufacturing, Database systems journal, IV, 3, 10-19.
Qi Q., Tao F. (2018), Digital twin and Big data towards smart Manufacturing and Industry 4.0: 360 Degree Comparison. IEEE Access, Vol VI, pp.3585-3593.
Ramakrishnan R., Baskar S., John D.R., Pavan K., Balaji K.S., Karthick K., Peng L., Mitica M., Spiro M., Rogério R., Sharman N., Zee X., Youssef B., Chris D., Draves R., Shrikant N.S., Shankar S., Atul S., Sun S., Ramarathnam V. (2017), Azure Data Lake Store: A Hyperscale Distributed File Service for Big Data Analytics, Proceedings of the 2017 ACM International conference on management of Data. Chicago, Illinois, USA.
Rao V. (2018), Extracting dark data, https://developer.ibm.com/articles/ba-data-becomes-knowledge-3/.
Reinsel D., Gantz J., Rydning j. (2018), The digitization of the world – From edge to core, https://www.seagate.com/files/www-content/our-story/trends/files/idc-seagate-dataage-whitepaper.pdf.
Ren S., Zhang Y., Liu Y., Tomohiko S., Donald H. (2019), A comprehensive review of Big data analytics throughout product lifecycle to support sustainable smart manufacturing: a framework, challenges and future research directions, Journal of cleaner production, CCX, 1343-1365.
Schembera B., Durán J.M. (2019), Dark data as the new challenge for Big data science and the introduction of the scientific Data Officer, Philosophy & Technology, 1-23, https://doi.org/10.1007/s13347-019-00346-x.
Sivarajah U., Kamal M.M., Zahir I., Weerakkody V. (2016), Critical analysis of Big data challenges and analytical methods, Journal of Business Research, Brunel university London, Brunel Business School, UB8 3PH, United Kingdom.
Solaimani M., Mohammed I., Latifur K., Bhavani T., Burton I.J. (2014), Spark-based anomaly detection over multi-source VMware performance data in real-time, IEEE Symposium on Computational Intelligence in Cyber Security (CICS), Orlando, FL, USA.
Trajanov D., Zdraveski V., Stojanov R., Kocarev L. (2018), Dark Data in Internet of Things (IoT): Challenges and Opportunities, Proceedings of the 7th small systems simulation symposium.
Trice A. (2015), The Future of Cognitive Computing, https://www.ibm.com/blogs/cloud-archive/2015/11/future-of-cognitive-computing/.
Zhang C., Shin J., Ré C., Cafarella M., Niu F. (2016), Extracting databases from Dark data with DeepDive, Proceedings of the 2016 International Conference on Management of Data, 847-859. http://dx.doi.org/10.1145/2882903.2904442.
Per informazioni sull’acquisto di copie e abbonamenti scrivi a daniela.bobbiese@este.it (tel. 02.91434400)